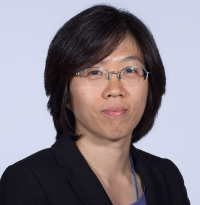
Professor Jitkomut Songsiri, Ph.D.
ศ. ดร.จิตโกมุท ส่งศิริ
Education
- Ph.D. Electrical Engineering, University of California, Los Angeles, U.S.A, 2010
- M.Eng. Electrical Engineering, Chulalongkorn University, Thailand, 2002
- B.Eng. Electrical Engineering, Chulalongkorn University, Thailand, 1999
Email: Jitkomut.s@chula.ac.th
Research Interest
- system modelling
- statistical learning
- non-smooth optimization
- sparse system identification
- sparse optimization
- solar power forecasting
- brain connectivity
- Granger causality
- causality learning in time series
Research Cluster
Boonyakitanont, P; Lek-uthai, A; Chomtho, K; Songsiri, J
A review of feature extraction and performance evaluation in epileptic seizure detection using EEG Journal Article
In: Biomedical Signal Processing and Control, vol. 57, 2020, ISSN: 17468094, (cited By 26).
@article{Boonyakitanont2020,
title = {A review of feature extraction and performance evaluation in epileptic seizure detection using EEG},
author = {P Boonyakitanont and A Lek-uthai and K Chomtho and J Songsiri},
url = {https://www.scopus.com/inward/record.uri?eid=2-s2.0-85076787553&doi=10.1016%2fj.bspc.2019.101702&partnerID=40&md5=501098aa4e3f116945d2c9b69745f424},
doi = {10.1016/j.bspc.2019.101702},
issn = {17468094},
year = {2020},
date = {2020-01-01},
journal = {Biomedical Signal Processing and Control},
volume = {57},
publisher = {Elsevier Ltd},
abstract = {Since the manual detection of electrographic seizures in continuous electroencephalogram (EEG) monitoring is very time-consuming and requires a trained expert, attempts to develop automatic seizure detection are diverse and ongoing. Machine learning approaches are intensely being applied to this problem due to their ability to classify seizure conditions from a large amount of data, and provide pre-screened results for neurologists. Several features, data transformations, and classifiers have been explored to analyze and classify seizures via EEG signals. In the literature, some jointly-applied features used in the classification may have shared similar contributions, making them redundant in the learning process. Therefore, this paper aims to comprehensively summarize feature descriptions and their interpretations in characterizing epileptic seizures using EEG signals, as well as to review classification performance metrics. To provide meaningful information of feature selection, we conducted an experiment to examine the quality of each feature independently. The Bayesian error and non-parametric probability distribution estimation were employed to determine the significance of the individual features. Moreover, a redundancy analysis using a correlation-based feature selection was applied. The results showed that the following features – variance, energy, nonlinear energy, and Shannon entropy computed on a raw EEG signal, as well as variance, energy, kurtosis, and line length calculated on wavelet coefficients – were able to significantly capture the seizures. When compared with a baseline method of classifying all epochs as normal, an improvement of 4.77–13.51% in the Bayesian error was obtained. © 2019 Elsevier Ltd},
note = {cited By 26},
keywords = {},
pubstate = {published},
tppubtype = {article}
}
Pruttiakaravanich, A; Songsiri, J
Convex formulation for regularized estimation of structural equation models Journal Article
In: Signal Processing, vol. 166, 2020, ISSN: 01651684, (cited By 3).
@article{Pruttiakaravanich2020,
title = {Convex formulation for regularized estimation of structural equation models},
author = {A Pruttiakaravanich and J Songsiri},
url = {https://www.scopus.com/inward/record.uri?eid=2-s2.0-85069879723&doi=10.1016%2fj.sigpro.2019.107237&partnerID=40&md5=e1a86a70bfe5b9adec72201d9ca8b7e9},
doi = {10.1016/j.sigpro.2019.107237},
issn = {01651684},
year = {2020},
date = {2020-01-01},
journal = {Signal Processing},
volume = {166},
publisher = {Elsevier B.V.},
abstract = {Path analysis is a model class of structural equation modeling (SEM), which it describes causal relations among measured variables in the form of a multiple linear regression. This paper presents two estimation formulations, one each for confirmatory and exploratory SEM, where a zero pattern of the estimated path matrix can explain a causality structure of the variables. The original nonlinear equality constraints of the model parameters were relaxed to an inequality, allowing the transformation of the original problem into a convex framework. A regularized estimation formulation was then proposed for exploratory SEM using an l1-type penalty of the path coefficient matrix. Under a condition on problem parameters, our optimal solution is low rank and provides a useful solution to the original problem. Proximal algorithms were applied to solve our convex programs in a large-scale setting. The performance of this approach was demonstrated in both simulated and real data sets, and in comparison with an existing method. When applied to two real applications (learning causality among climate variables and examining brain connectivity in autism patients using fMRI time series from ABIDE data set) the findings could explain known relationships among environmental variables and discern known and new brain connectivity differences, respectively. © 2019 Elsevier B.V.},
note = {cited By 3},
keywords = {},
pubstate = {published},
tppubtype = {article}
}
Pipattanasomporn, M; Chitalia, G; Songsiri, J; Aswakul, C; Pora, W; Suwankawin, S; Audomvongseree, K; Hoonchareon, N
CU-BEMS, smart building electricity consumption and indoor environmental sensor datasets Journal Article
In: Scientific Data, vol. 7, no. 1, 2020, ISSN: 20524463, (cited By 2).
@article{Pipattanasomporn2020,
title = {CU-BEMS, smart building electricity consumption and indoor environmental sensor datasets},
author = {M Pipattanasomporn and G Chitalia and J Songsiri and C Aswakul and W Pora and S Suwankawin and K Audomvongseree and N Hoonchareon},
url = {https://www.scopus.com/inward/record.uri?eid=2-s2.0-85088245691&doi=10.1038%2fs41597-020-00582-3&partnerID=40&md5=f4429e29617970d1a17b92a27f26ec05},
doi = {10.1038/s41597-020-00582-3},
issn = {20524463},
year = {2020},
date = {2020-01-01},
journal = {Scientific Data},
volume = {7},
number = {1},
publisher = {Nature Research},
abstract = {This paper describes the release of the detailed building operation data, including electricity consumption and indoor environmental measurements, of the seven-story 11,700-m2 office building located in Bangkok, Thailand. The electricity consumption data (kW) are that of individual air conditioning units, lighting, and plug loads in each of the 33 zones of the building. The indoor environmental sensor data comprise temperature (°C), relative humidity (%), and ambient light (lux) measurements of the same zones. The entire datasets are available at one-minute intervals for the period of 18 months from July 1, 2018, to December 31, 2019. Such datasets can be used to support a wide range of applications, such as zone-level, floor-level, and building-level load forecasting, indoor thermal model development, validation of building simulation models, development of demand response algorithms by load type, anomaly detection methods, and reinforcement learning algorithms for control of multiple AC units. © 2020, The Author(s).},
note = {cited By 2},
keywords = {},
pubstate = {published},
tppubtype = {article}
}
de Steen, F Van; Faes, L; Karahan, E; Songsiri, J; Valdes-Sosa, P A; Marinazzo, D
Critical Comments on EEG Sensor Space Dynamical Connectivity Analysis Journal Article
In: Brain Topography, vol. 32, no. 4, pp. 643-654, 2019, ISSN: 08960267, (cited By 38).
@article{VandeSteen2019,
title = {Critical Comments on EEG Sensor Space Dynamical Connectivity Analysis},
author = {F Van de Steen and L Faes and E Karahan and J Songsiri and P A Valdes-Sosa and D Marinazzo},
url = {https://www.scopus.com/inward/record.uri?eid=2-s2.0-85000384188&doi=10.1007%2fs10548-016-0538-7&partnerID=40&md5=b72c16c9b4c35c1f844c5c1769c8a98f},
doi = {10.1007/s10548-016-0538-7},
issn = {08960267},
year = {2019},
date = {2019-01-01},
journal = {Brain Topography},
volume = {32},
number = {4},
pages = {643-654},
publisher = {Springer New York LLC},
abstract = {Many different analysis techniques have been developed and applied to EEG recordings that allow one to investigate how different brain areas interact. One particular class of methods, based on the linear parametric representation of multiple interacting time series, is widely used to study causal connectivity in the brain. However, the results obtained by these methods should be interpreted with great care. The goal of this paper is to show, both theoretically and using simulations, that results obtained by applying causal connectivity measures on the sensor (scalp) time series do not allow interpretation in terms of interacting brain sources. This is because (1) the channel locations cannot be seen as an approximation of a source’s anatomical location and (2) spurious connectivity can occur between sensors. Although many measures of causal connectivity derived from EEG sensor time series are affected by the latter, here we will focus on the well-known time domain index of Granger causality (GC) and on the frequency domain directed transfer function (DTF). Using the state-space framework and designing two simulation studies we show that mixing effects caused by volume conduction can lead to spurious connections, detected either by time domain GC or by DTF. Therefore, GC/DTF causal connectivity measures should be computed at the source level, or derived within analysis frameworks that model the effects of volume conduction. Since mixing effects can also occur in the source space, it is advised to combine source space analysis with connectivity measures that are robust to mixing. © 2016, Springer Science+Business Media New York.},
note = {cited By 38},
keywords = {},
pubstate = {published},
tppubtype = {article}
}
Raksasri, N; Songsiri, J
Guaranteed stability of autoregressive models with granger causality learned from Wald tests Journal Article
In: Engineering Journal, vol. 21, no. 6 Special Issue, pp. 23-36, 2017, ISSN: 01258281, (cited By 2).
@article{Raksasri2017,
title = {Guaranteed stability of autoregressive models with granger causality learned from Wald tests},
author = {N Raksasri and J Songsiri},
url = {https://www.scopus.com/inward/record.uri?eid=2-s2.0-85033580175&doi=10.4186%2fej.2017.21.6.23&partnerID=40&md5=8eec07d74cc7bf75272272b9f2c0e6c6},
doi = {10.4186/ej.2017.21.6.23},
issn = {01258281},
year = {2017},
date = {2017-01-01},
journal = {Engineering Journal},
volume = {21},
number = {6 Special Issue},
pages = {23-36},
publisher = {Chulalongkorn University 1},
abstract = {This paper aims to explain relationships between time series by using the Granger causality (GC) concept through autoregressive (AR) models and to assure the model stability. Examining such GC relationship is performed on the model parameters using the Wald test and the model stability is guaranteed by the infinity-norm constraint on the dynamic matrix of the AR process. The proposed formulation is a least-squares estimation with Granger causality and stability constraints which is a convex program with a quadratic objective subject to linear equality and inequality norm constraints. We show by simulations that various typical factors could lead to unstable estimated models when using an unconstrained method. Estimated models from our approach are guaranteed to be stable but the model fitting error could be conservatively increased due to the selected stability condition. © 2017, Chulalongkorn University 1. All Rights Reserved.},
note = {cited By 2},
keywords = {},
pubstate = {published},
tppubtype = {article}
}
Pruttiakaravanich, A; Songsiri, J
A review on dependence measures in exploring brain networks from fMRI data Journal Article
In: Engineering Journal, vol. 20, no. 3, pp. 208-233, 2016, ISSN: 01258281, (cited By 1).
@article{Pruttiakaravanich2016a,
title = {A review on dependence measures in exploring brain networks from fMRI data},
author = {A Pruttiakaravanich and J Songsiri},
url = {https://www.scopus.com/inward/record.uri?eid=2-s2.0-84983548327&doi=10.4186%2fej.2016.20.3.207&partnerID=40&md5=4cac3d80c6c12238956165d2cc422c0c},
doi = {10.4186/ej.2016.20.3.207},
issn = {01258281},
year = {2016},
date = {2016-01-01},
journal = {Engineering Journal},
volume = {20},
number = {3},
pages = {208-233},
publisher = {Chulalongkorn University},
abstract = {Functional magnetic resonance imaging (fMRI) technique allows us to capture activities occurring in a human brain via signals related to cerebral blood flow, oxygen metabolism and blood volume, known as BOLD (blood oxygen level-dependent) signals. Exploring relationships between brain regions inside human brains from fMRI data is an active and challenging research topic. Relationships or associations between brain regions are commonly referred to as brain connectivity or brain network. This connectivity can be divided into three groups; (i) structural connectivity representing physically anatomical connections between regions, (ii) the functional connectivity which describes the statistical information among brain regions and (iii) the effective connectivity which specifies how one region interacts with others by a causal model. This survey paper provides a review on learning brain connectivities via fMRI data where detailed mathematical definitions of dependence measures widely-used for functional and effective connectivities are described. These measures include correlation, partial correlation, coherence, partial coherence, directed coherence, partial directed coherence, Granger causality, and other concepts such as dynamical causal modeling or structural equation modeling. Interpretation and relations of these measures as well as relevant estimation techniques that are widely used in the problems of fMRI modeling are summarized in this paper. © 2016, Chulalongkorn University 1. All rights reserved.},
note = {cited By 1},
keywords = {},
pubstate = {published},
tppubtype = {article}
}