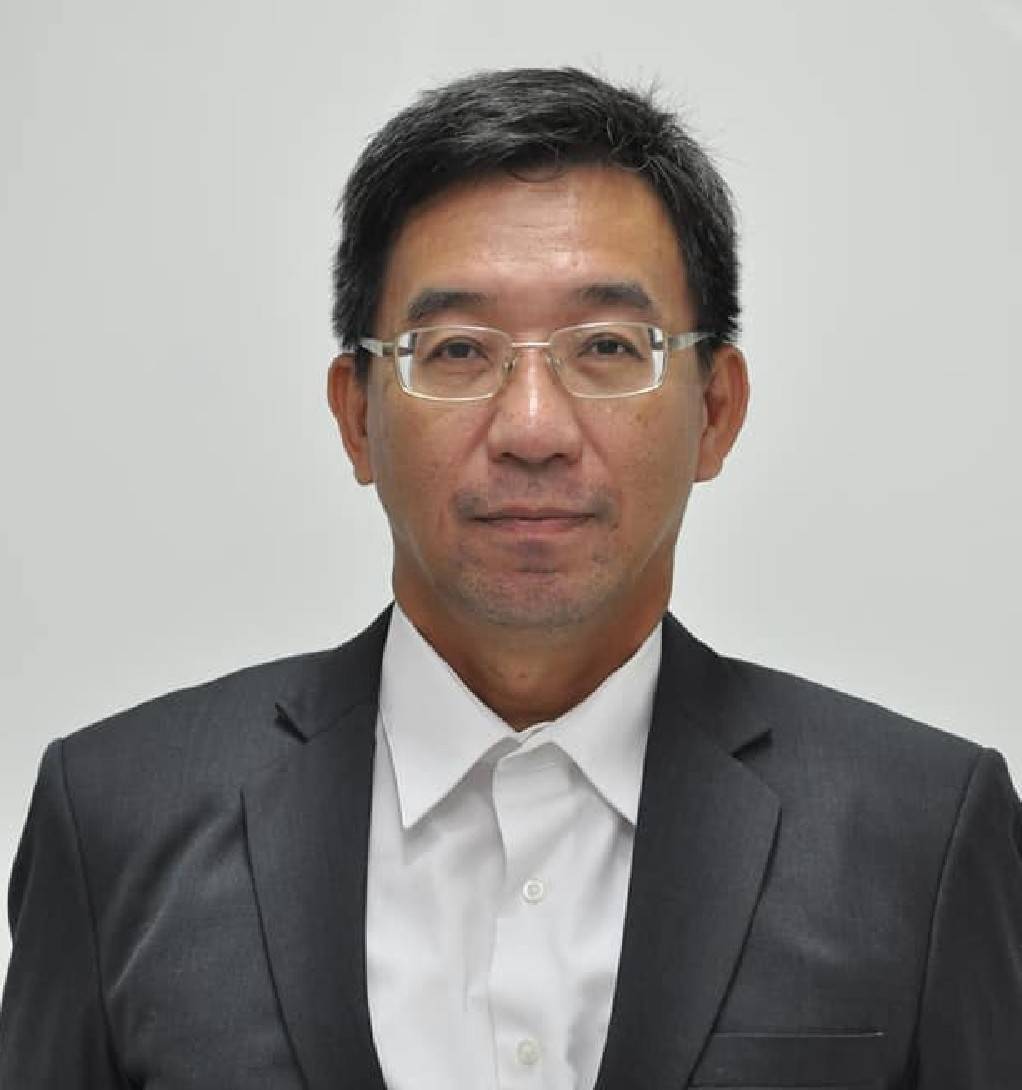
Assistant Professor Widhyakorn Asdornwised, Ph.D.
ผศ. ดร.วิทยากร อัศดรวิเศษ
Education
- Ph.D. Electrical Engineering, Chulalongkorn University, Thailand.
- M.Eng. Electrical Engineering, Chulalongkorn University, Thailand.
- B.Eng. Electrical Engineering, Kasetsart Univerity, Thailand.
Email: Widhyakorn.A@chula.ac.th
Research Interest
- 5G and Beyonds
- 5G Private Networks
- Signal Processing for Communications
- Statistical Inference and Deep Learning
- Sparse Representation
- Digital Signal Processing, and Image Processing
Research Cluster
Le, N T; Asdornwised, W; Chaitusaney, S; Benjapolakul, W
Application of the mixed effects model for analysing photovoltaic datasets and interpreting into meaningful insights Journal Article
In: IET Generation, Transmission and Distribution, 2021, ISSN: 17518687, (cited By 0).
@article{Le2021,
title = {Application of the mixed effects model for analysing photovoltaic datasets and interpreting into meaningful insights},
author = {N T Le and W Asdornwised and S Chaitusaney and W Benjapolakul},
url = {https://www.scopus.com/inward/record.uri?eid=2-s2.0-85102276868&doi=10.1049%2fgtd2.12160&partnerID=40&md5=396986630bf342b005b547783065c238},
doi = {10.1049/gtd2.12160},
issn = {17518687},
year = {2021},
date = {2021-01-01},
journal = {IET Generation, Transmission and Distribution},
publisher = {John Wiley and Sons Inc},
abstract = {Energy power studies commonly apply the analysis of variance (ANOVA) method in data analysis context to find the significant difference among many compared groups or to evaluate the impact of an influential factor. However, most of the datasets in these studies are time series data or longitudinal data, which are collected from the same object over some periods. Therefore, violating the independent assumption of ANOVA is the error commonly made. This leads to the misinterpretation of the comparison tests. In this article, this problem is solved by providing the application of mixed effects model (MEM) as a viable alternative to the ANOVA. In detail, two models based on MEM are proposed to analyse the time series data of micro-inverter PV stations located in Concord city, Massachusetts, USA. In the first scenario, the average models are implemented to compare the seasonal variation of monthly yield taking into account the effects of shading conditions and different orientations. In the second scenario, the linear regression model based on MEM is implemented to estimate and compare the decline rate in a 6-year period of PV stations. The analysis results have shown that the models based on MEM performs better than ANOVA in interpreting raw time-series dataset into meaningful insights. © 2021 The Authors. IET Generation, Transmission & Distribution published by John Wiley & Sons Ltd on behalf of The Institution of Engineering and Technology},
note = {cited By 0},
keywords = {},
pubstate = {published},
tppubtype = {article}
}
Kittisuwan, P; Marukatat, S; Chanwimaluang, T; Asdornwised, W
Image denoising employing two-sided gamma random vectors with cycle-spinning in wavelet domain Journal Article
In: ECTI Transactions on Electrical Engineering, Electronics, and Communications, vol. 9, no. 2, pp. 255-263, 2011, ISSN: 16859545, (cited By 2).
@article{Kittisuwan2011,
title = {Image denoising employing two-sided gamma random vectors with cycle-spinning in wavelet domain},
author = {P Kittisuwan and S Marukatat and T Chanwimaluang and W Asdornwised},
url = {https://www.scopus.com/inward/record.uri?eid=2-s2.0-80055087600&partnerID=40&md5=4c0c264fb9f6f81626749a24878b1809},
issn = {16859545},
year = {2011},
date = {2011-01-01},
journal = {ECTI Transactions on Electrical Engineering, Electronics, and Communications},
volume = {9},
number = {2},
pages = {255-263},
publisher = {ECTI Association},
abstract = {In this work, we present new Bayesian estimator for circularly-contoured Two-Sided Gamma random vector in additive white Gaussian noise (AWGN). This PDF is used in view of the fact that it is more peaked and the tails are heavier to be incorporated in the probabilistic modeling of the wavelet coefficients. One of the cruxes of the Bayesian image denoising methods is to estimate statistical parameters for a shrinkage function. We employ maximum a posterior (MAP) estimation to calculate local variances with Rayleigh density prior for local observed variances and Gaussian distribution for noisy wavelet coefficients. Several denoising methods (ProbShrink with redundant wavelet transform) using un-decimated wavelet transforms provide good results. The un-decimated wavelet transforms can also be viewed as applying an orthogonal wavelet transform to a set of shifted versions of the signal. This procedure was First suggested by Coifman and Donoho where they termed it cycle-spinning method. We apply cycle-spinning with orthogonal wavelet transforms in our work. The experimental results show that the proposed method yields good denoising results.},
note = {cited By 2},
keywords = {},
pubstate = {published},
tppubtype = {article}
}
Kittisuwan, P; Chanwimaluang, T; Marukatat, S; Asdornwised, W
Image and audio-speech denoising based on higher-order statistical modeling of wavelet coefficients and local variance estimation Journal Article
In: International Journal of Wavelets, Multiresolution and Information Processing, vol. 8, no. 6, pp. 987-1017, 2010, ISSN: 02196913, (cited By 15).
@article{Kittisuwan2010,
title = {Image and audio-speech denoising based on higher-order statistical modeling of wavelet coefficients and local variance estimation},
author = {P Kittisuwan and T Chanwimaluang and S Marukatat and W Asdornwised},
url = {https://www.scopus.com/inward/record.uri?eid=2-s2.0-78649620035&doi=10.1142%2fS0219691310003808&partnerID=40&md5=3d67fc954d0b4dccdbe8411d4cf0868f},
doi = {10.1142/S0219691310003808},
issn = {02196913},
year = {2010},
date = {2010-01-01},
journal = {International Journal of Wavelets, Multiresolution and Information Processing},
volume = {8},
number = {6},
pages = {987-1017},
abstract = {At first, this paper is concerned with wavelet-based image denoising using Bayesian technique. In conventional denoising process, the parameters of probability density function (PDF) are usually calculated from the first few moments, mean and variance. In the first part of our work, a new image denoising algorithm based on Pearson Type VII random vectors is proposed. This PDF is used because it allows higher-order moments to be incorporated into the noiseless wavelet coefficients' probabilistic model. One of the cruxes of the Bayesian image denoising algorithms is to estimate the variance of the clean image. Here, maximum a posterior (MAP) approach is employed for not only noiseless wavelet-coefficient estimation but also local observed variance acquisition. For the local observed variance estimation, the selection of noisy wavelet-coefficient model, either a Laplacian or a Gaussian distribution, is based upon the corrupted noise power where Gamma distribution is used as a prior for the variance. Evidently, our selection of prior is motivated by analytical and computational tractability. In our experiments, our proposed method gives promising denoising results with moderate complexity. Eventually, our image denoising method can be simply extended to audio/speech processing by forming matrix representation whose rows are formed by time segments of digital speech waveforms. This way, the use of our image denoising methods can be exploited to improve the performance of various audio/speech tasks, e.g., denoised enhancement of voice activity detection to capture voiced speech, significantly needed for speech coding and voice conversion applications. Moreover, one of the voice abnormality detections, called oropharyngeal dysphagia classification, is also required denoising method to improve the signal quality in elderly patients. We provide simple speech examples to demonstrate the prospects of our techniques. © 2010 World Scientific Publishing Company.},
note = {cited By 15},
keywords = {},
pubstate = {published},
tppubtype = {article}
}
Sanguansat, P; Asdornwised, W; Jitapunkul, S; Marukatat, S
Two-dimensional linear discriminant analysis of principle component vectors for face recognition Journal Article
In: IEICE Transactions on Information and Systems, vol. E89-D, no. 7, pp. 2164-2170, 2006, ISSN: 09168532, (cited By 17).
@article{Sanguansat2006b,
title = {Two-dimensional linear discriminant analysis of principle component vectors for face recognition},
author = {P Sanguansat and W Asdornwised and S Jitapunkul and S Marukatat},
url = {https://www.scopus.com/inward/record.uri?eid=2-s2.0-33747874461&doi=10.1093%2fietisy%2fe89-d.7.2164&partnerID=40&md5=f2c2e7961f6716b9b253485f6be26ee0},
doi = {10.1093/ietisy/e89-d.7.2164},
issn = {09168532},
year = {2006},
date = {2006-01-01},
journal = {IEICE Transactions on Information and Systems},
volume = {E89-D},
number = {7},
pages = {2164-2170},
publisher = {Institute of Electronics, Information and Communication, Engineers, IEICE},
abstract = {In this paper, we proposed a new Two-Dimensional Linear Discriminant Analysis (2DLDA) method, based on Two-Dimensional Principle Component Analysis (2DPCA) concept. In particular, 2D face image matrices do not need to be previously transformed into a vector. In this way, the spatial information can be preserved. Moreover, the 2DLDA also allows avoiding the Small Sample Size (SSS) problem, thus overcoming the traditional LDA. We combine 2DPCA and our proposed 2DLDA on the Two-Dimensional Linear Discriminant Analysis of principle component vectors framework. Our framework consists of two steps: first we project an input face image into the family of projected vectors via 2DPCA-based technique, second we project from these space into the classification space via 2DLDA-based technique. This does not only allows further reducing of the dimension of feature matrix but also improving the classification accuracy. Experimental results on ORL and Yale face database showed an improvement of 2DPCA-based technique over the conventional PCA technique. Copyright © 2006 The Institute of Electronics, Information and Communication Engineers.},
note = {cited By 17},
keywords = {},
pubstate = {published},
tppubtype = {article}
}
Asdornwised, W; Jitapunkul, S
Multiple description pattern analysis: Robustness to misclassification using local discriminant frame expansions Journal Article
In: IEICE Transactions on Information and Systems, vol. E88-D, no. 10, pp. 2296-2307, 2005, ISSN: 09168532, (cited By 0).
@article{Asdornwised2005,
title = {Multiple description pattern analysis: Robustness to misclassification using local discriminant frame expansions},
author = {W Asdornwised and S Jitapunkul},
url = {https://www.scopus.com/inward/record.uri?eid=2-s2.0-33645676775&doi=10.1093%2fietisy%2fe88-d.10.2296&partnerID=40&md5=c5fc44ae094a8b821125b1d77c7a4827},
doi = {10.1093/ietisy/e88-d.10.2296},
issn = {09168532},
year = {2005},
date = {2005-01-01},
journal = {IEICE Transactions on Information and Systems},
volume = {E88-D},
number = {10},
pages = {2296-2307},
publisher = {Institute of Electronics, Information and Communication, Engineers, IEICE},
abstract = {In this paper, a source coding model for learning multiple concept descriptions of data is proposed. Our source coding model is based on the concept of transmitting data over multiple channels, called multiple description (MD) coding. In particular, frame expansions have been used in our MD coding models for pattern classification. Using this model, there are several interesting properties within a class of multiple classifier algorithms that share with our proposed scheme. Generalization of the MD view under an extension of local discriminant basis towards the theory of frames allows the formulation of a generalized class of low-complexity learning algorithms applicable to high-dimensional pattern classification. To evaluate this approach, performance results for automatic target recognition (ATR) are presented for synthetic aperture radar (SAR) images from the MSTAR public release data set. From the experimental results, our approach outperforms state-of-the-art methods such as conditional Gaussian signal model, Adaboost, and ECOC-SVM. Copyright © 2005 The Institute of Electronics, Information and Communication Engineers.},
note = {cited By 0},
keywords = {},
pubstate = {published},
tppubtype = {article}
}
Asdornwised, W; Jitapunkul, S
Automatic target recognition using multiple description coding models for multiple classifier systems Journal Article
In: Lecture Notes in Computer Science (including subseries Lecture Notes in Artificial Intelligence and Lecture Notes in Bioinformatics), vol. 2709, pp. 336-345, 2003, ISSN: 03029743, (cited By 4).
@article{Asdornwised2003,
title = {Automatic target recognition using multiple description coding models for multiple classifier systems},
author = {W Asdornwised and S Jitapunkul},
editor = {Roli F Windeatt T.},
url = {https://www.scopus.com/inward/record.uri?eid=2-s2.0-21144453216&doi=10.1007%2f3-540-44938-8_34&partnerID=40&md5=489a57d34ed7ee8d6338f1f2fd1a8875},
doi = {10.1007/3-540-44938-8_34},
issn = {03029743},
year = {2003},
date = {2003-01-01},
journal = {Lecture Notes in Computer Science (including subseries Lecture Notes in Artificial Intelligence and Lecture Notes in Bioinformatics)},
volume = {2709},
pages = {336-345},
publisher = {Springer Verlag},
abstract = {In this paper, we proposed a new multiple classifier system (MCS) based on multiple description coding (MDC) models. Our proposed method was inspired from the framework of transmitting data over heterogeneous network, especially wireless network. In order to support the idea of MDC in pattern classification, parallels between transmission of concepts (hypothesis) and transmission of information through a noisy channel are addressed. Furthermore, preliminary surveys on the biological plausible of the MDC concepts are also included. One of the benefits of our approach is that it allows us to formulate a generalized class of signal processing based weak classification algorithms. This will be very applicable for MCS in high dimensional classification problems, such as image recognition. Performance results for automatic target recognition are presented for synthetic aperture radar (SAR) images from the MSTAR public release data set. © Springer-Verlag Berlin Heidelberg 2003.},
note = {cited By 4},
keywords = {},
pubstate = {published},
tppubtype = {article}
}